As data visualizations, word clouds have a place in brainstorming sessions and creating stylish infographics. Yet when strategists and decision-makers really want to get to the bottom of a business issue or understand what’s on customers’ minds, they’ll have to level up with innovative data analysis.
Word clouds vs. text analytics
A quick and easy visualization option for highlighting the frequency of key words within text-based data, word clouds can spark ideas and conversations. Looked at from the perspective of AI text analytics tools like Keatext, which just as quickly and easily analyze qualitative and verbatim data, word clouds fall far short of providing insights into that data.
In an article about exploring historical data, a word cloud based on the text of War and Peace demonstrates that though word clouds highlight key words and main topics, they lack the vital context to make sense of the narrative, the nuances of characters and what is culturally and politically at play in the book’s main themes.
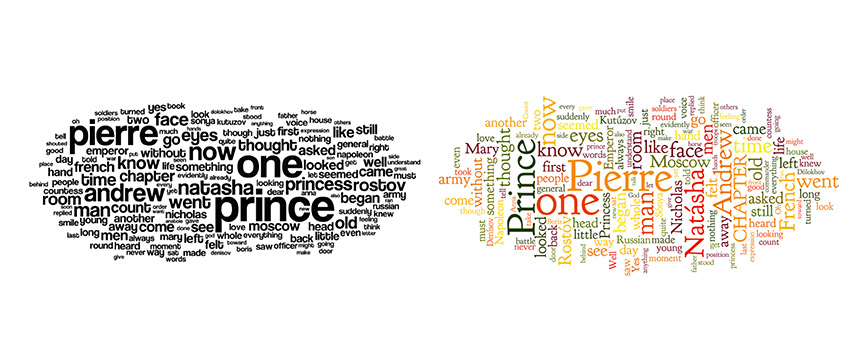
Rather than providing accuracy and insight into the text-based data, the word cloud picks out the most used words and ranks them by size. For someone who hasn’t read War and Peace, the tool isn’t of much use in understanding the novel. Analyzing the novel using a text analytics tool, however, offers more meaningful and complex insight into the story, its themes and relationships.
In the process of a few different word cloud tools on a digital text of War and Peace, the three major strengths and associated weaknesses of word clouds stand out:
- While word clouds are easy to create, taking as little as two minutes with a free word cloud tool and available text-based data, they are also full of blind spots, especially for a user looking for any deeper research or argument.
- Because word clouds rank word value by size to create an easy-to-grasp image, they are simple to understand. However, since the visibility of words changes across the different cloud styles, especially for smaller words, the value placed on text is often inaccurate.
- A word cloud typically piques interest by being a casual and visually appealing way to visualize data, but their attractiveness can’t make up for a lack of context: word clouds simply can’t decipher the relationship between words or topics.
Why brands need text analytics, not word clouds
Today, companies are faced with a truly enormous amount of data – customer data alone comes from multiple sources now, from tried-and-true NPS surveys to customer reviews on major retail sites to conversational data from chatbots and customer service interactions. What companies really need to compete in this data-rich climate are tools for data analysis, tools that provide meaningful context and actionable insights.
Uploading the entire text of War and Peace into Keatext’s AI-powered text analytics platform results in more accuracy, context and even sentiment.
While word clouds have their strengths and place in summarizing some kinds of data, they aren’t capable of drawing out further value in it. Uploading the entire text of War and Peace into Keatext’s AI-powered text analytics platform results in more accuracy, context and even sentiment. Analyzing half a million words in less than 15 minutes, Keatext processes this famed work of literature just as it would analyze thousands of product reviews from a retail website’s feedback portal, finding top topic results and much more.
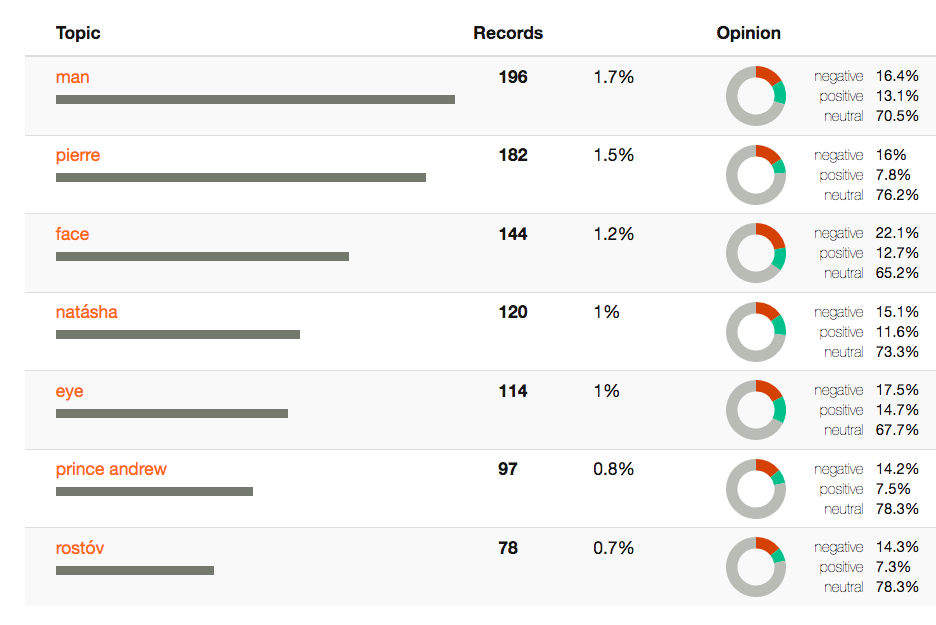
For example, Keatext’s top word, “man”, barely showed up in word clouds, yet ranked much higher thanks to the AI tool’s ability to automatically bundle similar terms (such as “man” and “men”). While Keatext displayed “Prince Andrew” as one topic, the word cloud seemed to suggest that “Pierre” and “prince” go hand in hand – able to provide context to both words, Keatext showed the real relation between them.
In addition to providing context, Keatext includes a sentiment analysis feature. In its analysis of the novel, Keatext found Natasha to be quite balanced as a character, while Pierre and Andrew are slightly more negative. In essence, Keatext analyzed the text as if it were analyzing the words of real people to determine their behaviours and needs – much like it would with a brand’s own customers.
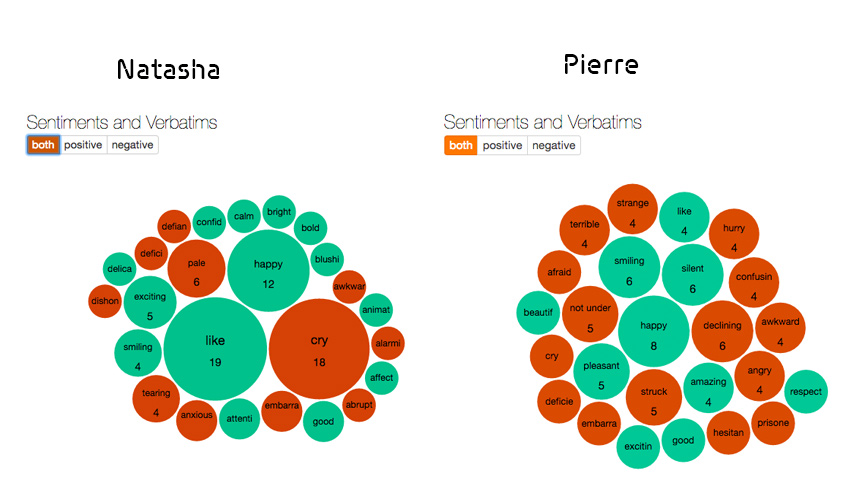
Learn more about text analytics
Read our complete guide to text analytics here.
The business use case for text analytics
Keatext’s AI data analysis is about prioritizing the valuable insights within an organization’s data. As data analysis becomes integral to tracking KPIs and meeting key objectives, the stronger the insights, the more competitive the business advantage. In our current climate, new AI platforms are the strongest tool for uncovering insights within customers’ own words.
Keatext’s advanced features offer something a word cloud truly can’t, including data collection from multiple sources, streamlined data integration, and reconfiguring the Keatext dashboard with Keatext’s development and analysis team.
Comprehensive data analysis that provides a high level of insight is able to help uncover topics and problems that might never have been recognized. From there, insights can be applied to CX strategies that span the customer journey, new marketing campaigns and assessment of previous ones, day-to-day operations and employee management. They can contribute to new directions and solutions to specific issues, from how customers want to shop to what employees need to maintain retention. After all, if a company doesn’t understand what’s going on with its customers, clients or employees, it can neither fix those problems or offer the right solutions.
While Keatext’s web-based AI text analytics platform can be easily used on its own by CX strategists, marketing teams, operations managers and other decision makers, Keatext’s advanced features offer something a word cloud truly can’t. That includes data collection from multiple sources and streamlined data integration with Zendesk, Surveymonkey, Salesforce, Intercom or any other of our 400+ integrations.
What was once the biggest strength of word clouds – data visualization – has progressed into a new realm in the hands of powerful analytics technology. Tied directly to both analyzed data and insights, Keatext’s visualization capabilities highlight topics, sentiments and any combination of factors that a company requires. With each analysis comes insights tailor-made to inspire action and decision making that can make a difference to a company’s bottom line.